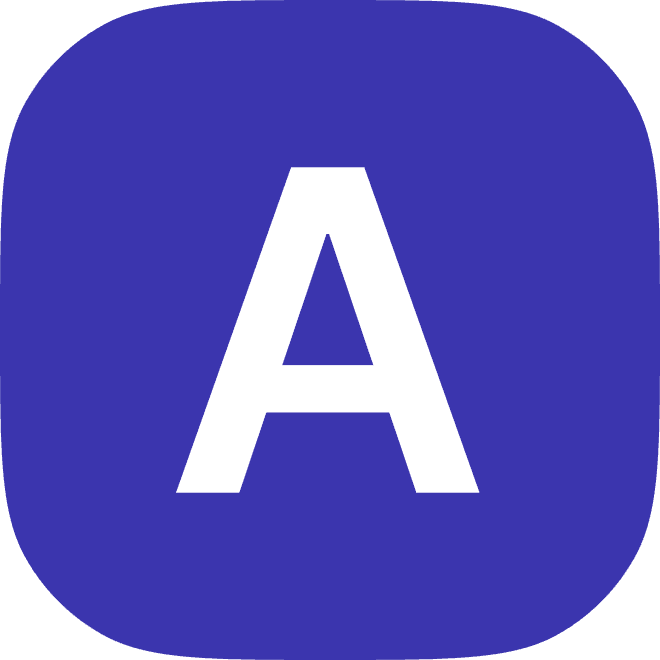
2023
Unlocking Potential: 5 Senior Data Scientist Behavioral Interview Questions That Work
Are you aspiring to become a senior data scientist? Do you want to ace your behavioral interview and showcase your skills and expertise? Look no further! In this article, we will provide you with 5 powerful behavioral interview questions specifically designed for senior data scientists.
As the field of data science continues to evolve, employers are increasingly focusing on behavioral interviews to assess a candidate's suitability for senior positions. These interviews aim to delve into your past experiences, problem-solving abilities, and decision-making skills.
Why should you care about these behavioral interview questions? Well, acing your interview can open doors to exciting career opportunities and help you land that dream job as a senior data scientist. By preparing for these questions, you can confidently demonstrate your capabilities and stand out from the competition.
So, what are these 5 senior data scientist behavioral interview questions that work? Let's dive in and explore them in detail:
1. Tell me about a challenging data science project you worked on and how you overcame obstacles.
2. Describe a situation where you had to make a major decision based on incomplete or ambiguous data.
3. Can you provide an example of a time when you had to explain complex data science concepts to non-technical stakeholders?
4. Share a time when you had to collaborate with a multidisciplinary team to achieve a data-driven solution.
5. How do you approach ethical considerations in data science projects?
By addressing these behavioral interview questions effectively, you can showcase your problem-solving skills, communication abilities, collaboration experience, decision-making capabilities, and ethical mindset - all essential qualities for a successful senior data scientist.
So, let's get started and unlock your potential as a senior data scientist!
The Importance of Behavioral Interviewing in Data Science
When it comes to hiring senior data scientists, technical skills alone are not enough to ensure success. Data science is a multidisciplinary field that requires a delicate balance of technical acumen, problem-solving abilities, teamwork, and effective communication. This is where behavioral interviewing comes into play.
Behavioral interviews are designed to go beyond a candidate's resume and technical expertise, providing insights into their behavioral traits, decision-making processes, and interpersonal skills. By asking the right questions, hiring managers can uncover a candidate's true potential and determine how well they would fit within their team.
Data science projects often involve complex problem-solving and collaboration with cross-functional teams. It's crucial to assess a candidate's ability to not only analyze data but also communicate their findings to non-technical stakeholders. Behavioral interviews allow recruiters to evaluate a candidate's problem-solving skills, teamwork, and communication abilities, ensuring they possess the right combination of technical and soft skills.
However, the unique challenges of data science recruitment make it essential to dig deeper during the interview process. Senior data scientists are expected to lead projects, mentor junior team members, and drive innovation within the organization. Behavioral interviewing provides a holistic view of a candidate's capabilities, helping hiring managers identify those who can thrive in this dynamic and demanding field.
Now, let's explore the five key behavioral interview questions that can unlock a senior data scientist's potential.
Question 1: Describe a Situation Where You Had to Make a Difficult Data-Driven Decision
When it comes to evaluating senior data scientists, one of the most critical aspects is their ability to make sound data-driven decisions. This question serves as a powerful tool to assess their data interpretation skills and decision-making process. By asking candidates to describe a situation where they had to make a difficult data-driven decision, you can gain valuable insights into their problem-solving abilities and their approach to risk and uncertainty.
Real-world examples of challenging data-driven decisions can provide a wealth of information about a candidate's capabilities. For instance, consider a scenario where a data scientist had to determine whether to launch a new product based on market analysis and customer feedback. This decision required them to analyze complex datasets, identify trends, and weigh the potential risks and benefits. By delving into such examples, you can gauge the candidate's ability to navigate complex data and make informed decisions that align with the organization's goals.
Understanding a candidate's approach to risk and uncertainty is crucial in the field of data science. The ability to balance the potential gains and losses, assess the reliability of data sources, and make confident decisions in the face of uncertainty is what sets exceptional senior data scientists apart. By exploring their decision-making process in challenging situations, you can gain insights into their analytical thinking, judgment, and ability to handle high-pressure scenarios.
Asking this question not only allows you to evaluate a candidate's technical skills but also their ability to communicate their decision-making process effectively. A strong candidate will be able to articulate their methodology, explain the factors they considered, and justify their ultimate decision. This demonstrates their ability to not only make data-driven decisions but also convey their thought process to stakeholders who may not have a technical background.
By asking candidates to describe a difficult data-driven decision they have faced, you can uncover their problem-solving abilities, analytical thinking, and communication skills. This question provides a comprehensive view of a candidate's potential to excel in a senior data scientist role.
Question 2: Can You Share an Instance When You Had to Explain Complex Data to a Non-Technical Audience?
One of the key qualities that separates exceptional data scientists from the rest is their ability to effectively communicate complex information to non-technical stakeholders. In the fast-paced and ever-evolving field of data science, being able to translate complex data into actionable insights is crucial for driving informed decision-making and fostering collaboration within a team.
When asking this question, we are not just evaluating a candidate's communication skills, but also their empathy towards non-technical audiences. It's not enough to simply explain the data; it's important to do so in a way that is clear, concise, and easily understandable to individuals who may not have a technical background.
A candidate's response to this question should demonstrate their ability to simplify complex concepts without oversimplifying or losing important details. Look for candidates who can break down technical jargon into layman's terms, using relatable examples and analogies to bridge the gap between data science and everyday life.
Effective communication is not just about conveying information; it's about ensuring that the audience understands and can act upon that information. Look for candidates who can explain not just the what of the data, but also the so what and the now what. Can they clearly articulate the implications of the data and the actions that should be taken based on those insights?
Communication skills are essential for collaboration within a data science team. When team members can effectively explain their findings and ideas to others, it leads to more productive discussions and better decision-making. A candidate who excels at explaining complex data to non-technical stakeholders is more likely to foster a collaborative environment where diverse perspectives are valued and understood.
In summary, when asking candidates to share an instance when they had to explain complex data to a non-technical audience, look for:
Clarity: Can they simplify complex concepts without losing important details?
Simplicity: Do they use relatable examples and analogies to bridge the gap between technical and non-technical audiences?
Actionability: Can they clearly articulate the implications of the data and the actions that should be taken based on those insights?
Empathy: Do they consider the needs and understanding of the non-technical audience?
Collaboration: Are they able to foster effective collaboration and decision-making within a data science team through their communication skills?
Remember, effective communication is the cornerstone of successful data science projects. By asking this question, you can identify candidates who not only possess the technical skills needed for the role but also have the ability to effectively communicate their findings and insights to a wide range of stakeholders.
Next, let's explore another crucial behavioral interview question: Question 3: How Have You Handled Conflicting Opinions or Ideas in a Team Setting?
Question 3: How Have You Handled Conflicting Opinions or Ideas in a Team Setting?
In the fast-paced world of data science, collaboration and teamwork are essential for success. The ability to navigate conflicting opinions and ideas is crucial in fostering a harmonious and productive team environment. That's why asking candidates about their experiences in handling such situations is a key part of the behavioral interview process.
The importance of this question in understanding a candidate's teamwork abilities and conflict resolution skills.
When working on complex data science projects, teams often consist of individuals with diverse backgrounds, skill sets, and perspectives. Conflicting opinions and ideas are bound to arise, and a candidate's response to these challenges can provide valuable insights into their ability to collaborate effectively.
By asking this question, you can gauge a candidate's ability to navigate disagreements, find common ground, and work towards shared goals. Their response can reveal their communication skills, emotional intelligence, and problem-solving abilities, all of which are critical in a team setting.
How a candidate's response can reveal their capacity to balance different viewpoints and foster a collaborative environment.
A candidate's response to this question can shed light on their approach to conflict resolution and their ability to create a harmonious team dynamic. Look for candidates who can articulate how they actively listen to different perspectives, respect diverse viewpoints, and find common ground.
Effective candidates will demonstrate their capacity to facilitate open and respectful discussions, encouraging team members to share their ideas and concerns. They will also showcase their ability to synthesize differing opinions and guide the team towards consensus.
The relevance of this question to the often collaborative and multi-disciplinary nature of data science projects.
Data science projects are rarely solitary endeavors. They require collaboration among data scientists, engineers, domain experts, and stakeholders from various departments. The ability to handle conflicting opinions and ideas is crucial in such multi-disciplinary environments.
A candidate who has successfully navigated conflicting opinions in the past demonstrates their adaptability, empathy, and respect for others. These qualities are essential for fostering a collaborative environment where ideas can be freely shared, and innovation can thrive.
By asking this question, you can assess a candidate's interpersonal skills and their ability to work effectively in diverse teams. Look for candidates who can showcase their experiences in maintaining positive relationships, resolving conflicts, and building consensus.
As you evaluate candidates' responses to this question, consider how their experiences align with the specific needs and dynamics of your data science team. Look for individuals who can not only handle conflicting opinions but also leverage them to drive innovation and foster a collaborative culture.
Now that we've explored the importance of understanding how candidates handle conflicting opinions and ideas, let's move on to the next behavioral interview question that can unlock the potential of senior data scientists.
Question 4: Can You Discuss a Time When You Had to Learn a New Skill or Tool Quickly to Complete a Project?
Adaptability and continual learning are essential qualities for success in the ever-evolving field of data science. As technology advances at a rapid pace, data scientists must be able to quickly learn new skills and tools to stay ahead of the curve. This question is crucial in assessing a candidate's adaptability, eagerness to learn, and problem-solving abilities.
When asking this question, you are not just looking for a candidate who can learn new skills, but also someone who can effectively apply those skills to complete a project successfully. A candidate's response can provide valuable insights into their resourcefulness and commitment to continuous learning.
For example, imagine a scenario where a data scientist was assigned to a project that required using a new programming language or statistical modeling technique. The candidate's answer should demonstrate their ability to quickly grasp the fundamentals of the new skill or tool and apply it to solve the problem at hand.
Look for candidates who can articulate the specific steps they took to learn the new skill or tool. Did they seek out online resources, attend training programs, or collaborate with colleagues who had expertise in that area? The ability to proactively seek out learning opportunities and leverage available resources is a strong indicator of a candidate's commitment to growth.
Additionally, pay attention to how the candidate describes their problem-solving process during this learning experience. Did they encounter any challenges along the way? How did they overcome those challenges? A candidate who can navigate obstacles and adapt their approach demonstrates resilience and tenacity, qualities that are highly valuable in the data science field.
By asking this question, you are not only assessing a candidate's ability to learn new skills, but also their problem-solving abilities and their commitment to personal and professional growth. In a field as dynamic as data science, adaptability and rapid learning are prerequisites for success.
Why is Adaptability and Rapid Learning Essential in Data Science?
Data science is a rapidly evolving field, with new technologies, tools, and techniques emerging regularly. To stay ahead of the curve and deliver impactful insights, data scientists must be adaptable and quick learners.
Here are a few reasons why adaptability and rapid learning are essential in data science:
Keeping up with technological advancements: The field of data science is heavily reliant on technology. New tools and platforms are constantly being developed, and data scientists need to quickly learn and adapt to these advancements to leverage them effectively in their work.
Solving complex problems: Data science projects often involve solving complex problems that require a multidisciplinary approach. Being adaptable allows data scientists to explore different perspectives and methodologies, increasing the likelihood of finding innovative solutions.
Dealing with evolving data: Data is constantly changing in terms of volume, variety, and velocity. Data scientists need to adapt their approaches to handle different types of data and extract meaningful insights from it.
Remaining competitive in the job market: As the demand for data scientists continues to grow, the job market becomes increasingly competitive. Employers are looking for candidates who can quickly learn and apply new skills, as they can bring fresh perspectives and drive innovation within their organizations.
By assessing a candidate's adaptability and eagerness to learn through this question, you can ensure that you are selecting data scientists who have the potential to thrive in a rapidly changing environment.
The only constant in the field of data science is change. Data scientists must embrace adaptability and rapid learning to stay relevant and make a meaningful impact. - Dr. Maxine Foster
Related Resources:
For more insights into data science interview questions, you may find the following resources helpful:
These resources provide additional interview questions and insights specifically tailored to data science and analytics roles.
Question 5: Describe a Situation When a Project Did Not Go as Planned and How You Handled It
One of the most valuable qualities in a senior data scientist is the ability to navigate and overcome challenges. In the fast-paced world of data science, projects don't always go according to plan. That's why it's crucial to assess a candidate's resilience, problem-solving skills, and ability to learn from failure through this question.
A candidate's answer to this question can offer deep insights into their approach when faced with setbacks and unexpected circumstances. It reveals their adaptability, resourcefulness, and their capacity to maintain composure under pressure.
In the field of data science, projects are often complex and multifaceted. They require meticulous planning, collaboration, and the ability to navigate through uncharted territories. However, even with the most well-thought-out strategies, unforeseen obstacles can arise. By asking candidates to describe a situation when a project did not go as planned, you gain valuable insights into their problem-solving abilities and their capacity to pivot and find alternative solutions.
Resilience is a key trait in any successful data scientist. It's the ability to bounce back from setbacks and learn from failures. A candidate who can articulate how they handled a project that did not go as planned demonstrates their willingness to take ownership of challenges and find innovative ways to overcome them.
When evaluating a candidate's response, consider the following:
Adaptability: Did the candidate demonstrate the ability to adapt to unexpected changes in the project's scope or requirements?
Problem-solving skills: How did the candidate approach the problem? Did they employ creative thinking and analytical skills to identify potential solutions?
Learning from failure: Did the candidate reflect on the experience and extract valuable lessons from the project's shortcomings? Did they demonstrate a growth mindset?
Collaboration: How did the candidate involve their team members in finding solutions? Did they effectively communicate and coordinate efforts to mitigate the impact of the project's challenges?
By asking this question, you not only gain insights into a candidate's ability to handle adversity but also assess their potential to contribute to a collaborative and supportive team environment. It showcases their ability to learn from their mistakes, adapt to new circumstances, and drive continuous improvement.
Resilience and learning from failure are essential traits in the fast-paced, high-stakes world of data science. Projects can be complex, and unexpected hurdles can arise at any moment. A senior data scientist who can handle these challenges with grace and creativity is an invaluable asset to any team.
Continue the journey of unlocking the potential of senior data scientists by exploring the next section, where we address frequently asked questions about behavioral interviewing for this role.
Image Source: Unsplash
Conclusion
Behavioral interviewing is a powerful tool in unlocking the potential of senior data scientists. By asking the right questions, recruiters can gain valuable insights into a candidate's problem-solving skills, teamwork abilities, communication prowess, adaptability, and resilience. These qualities are crucial in the fast-paced, high-stakes world of data science, where professionals must navigate complex challenges and collaborate effectively to drive meaningful outcomes.
Throughout this article, we explored five key behavioral interview questions that can help assess a senior data scientist's potential:
Describe a Situation Where You Had to Make a Difficult Data-Driven Decision: This question provides insights into a candidate's data interpretation skills, decision-making process, and approach to risk and uncertainty.
Can You Share an Instance When You Had to Explain Complex Data to a Non-Technical Audience? This question evaluates a candidate's communication skills, empathy towards non-technical audiences, and ability to translate complex data into actionable insights.
How Have You Handled Conflicting Opinions or Ideas in a Team Setting? By answering this question, candidates demonstrate their teamwork abilities, conflict resolution skills, and capacity to foster a collaborative environment.
Can You Discuss a Time When You Had to Learn a New Skill or Tool Quickly to Complete a Project? This question assesses a candidate's adaptability, eagerness to learn, resourcefulness, and commitment to continual learning.
Describe a Situation When a Project Did Not Go as Planned and How You Handled It: This question evaluates a candidate's resilience, problem-solving skills, and ability to learn from failure, which are crucial qualities in the dynamic field of data science.
By incorporating these behavioral interview questions into the recruitment process, organizations can identify senior data scientists who possess the right combination of technical expertise and soft skills. These individuals have the potential to drive innovation, foster collaboration, and deliver impactful results within their teams.
So, whether you're a recruiter, hiring manager, or aspiring data scientist, remember the power of behavioral interviewing in unlocking potential. Use these questions as a guide to uncover the true capabilities of senior data scientists and build a team that can thrive in the ever-evolving landscape of data science.
Now, it's time to put these insights into action. Start incorporating these behavioral interview questions into your recruitment process and witness the transformative impact they can have on your team's success.
Unlock the potential of senior data scientists through effective behavioral interview questions, and unlock the future of your organization.
No more hurriedly scribbled notes. Aspect delivers clear, detailed and custom AI summaries of every interview, capturing the nuances that matter.
Learn how to improve your interviewing technique with personalized feedback based on your interactions.
End-to-end integration: Aspect seamlessly integrates with your existing ATS systems, providing a unified hiring solution.
Beatriz F
People Success Specialist
Absolutely game-changing for busy recruiters!
The summary, the Q&A feature and the ATS integration have boosted my productivity and lowered the context-switching stress, the analytics provided allowed for me and my team to have full visibility over our stats, and Aspect's team couldn't be more helpful, friendly and accessible!
Diane O
CEO
Aspect adds rocket fuel to the hiring process.
Aspect helps me hire faster & more efficiently. I can create short highlight reels to share quickly with my team & clients for faster decision making. Faster, more informed decisions using Aspect has led to faster, better hires!
Lana R
Recruiter